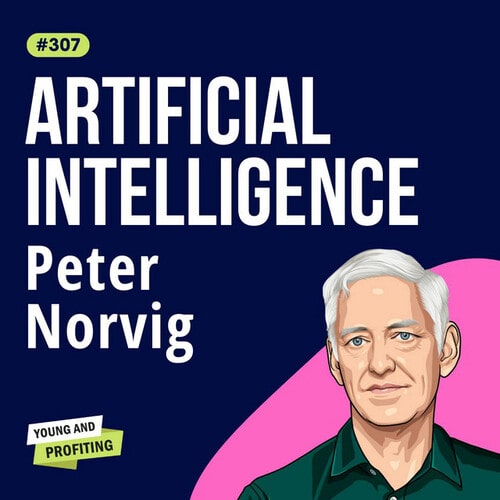
Peter Norvig: Simple Ways to Grow Your Business with AI | E307
Peter Norvig: Simple Ways to Grow Your Business with AI | E307
Peter Norvig is a leading AI expert, Stanford Fellow, and former Director of Research at Google, where he oversaw the development of transformative AI technologies. His contributions to AI and technology have earned him numerous accolades, including the NASA Exceptional Achievement Medal and the Berkeley Engineering Innovation Award.
In this episode, Hala and Peter will discuss:
– Peter’s transition from academia to the corporate world
– How AI is changing the way we live and work
– Practical ways entrepreneurs can leverage AI right now
– How AI is making learning more personalized
– Tips to stay competitive in an AI-driven market
– How AI can bridge skill gaps in the workforce
– Why we must maintain human control over AI
– The impact of automation on income inequality
– Why AI will generate more solopreneurs
– Ethical considerations of AI in society
– And other topics…
Peter Norvig is a computer scientist and a leading expert in artificial intelligence. He is a Fellow at Stanford’s Human-Centered AI Institute and a researcher at Google Inc. As Google’s Director of Research, Peter oversaw the evolution of search algorithms and built teams focused on groundbreaking advancements in machine translation, speech recognition, and computer vision. Earlier in his career, he led a team at NASA Ames that created autonomous software, which was a precursor to the Mars rovers. Also an influential educator, Peter co-authored the widely used textbook, Artificial Intelligence, which is taught in over 1,500 universities worldwide. His contributions to AI and technology have earned him numerous accolades, including the NASA Exceptional Achievement Medal and the Berkeley Engineering Innovation Award.
Connect With Peter:
Peter’s Profile: https://hai.stanford.edu/people/peter-norvig
Peter’s LinkedIn: https://www.linkedin.com/in/pnorvig/
Peter’s Facebook: https://www.facebook.com/peter.norvig
Resources Mentioned:
Peter’s Book, Artificial Intelligence: A Modern Approach: https://www.amazon.com/Artificial-Intelligence-Modern-Approach-3rd/dp/0136042597
Google AI Principles: https://ai.google/responsibility/principles/
LinkedIn Secrets Masterclass, Have Job Security For Life:
Use code ‘podcast’ for 30% off at yapmedia.io/course
Sponsored By:
Shopify – Sign up for a one-dollar-per-month trial period at youngandprofiting.co/shopify
Indeed – Get a $75 job credit at indeed.com/profiting
Found – Try Found for FREE at found.com/YAP
Rakuten – Start all your shopping at rakuten.com or get the Rakuten app to start saving today, your Cash Back really adds up!
Mint Mobile – To get a new 3-month premium wireless plan for just 15 bucks a month, go to mintmobile.com/profiting
Connectteam – Enjoy a 14-day free trial with no credit card needed. Open an account today at Connecteam.com
Working Genius – Get 20% off the $25 Working Genius assessment at WorkingGenius.com with code PROFITING at checkout
Top Deals of the Week: https://youngandprofiting.com/deals/
More About Young and Profiting
Download Transcripts – youngandprofiting.com
Get Sponsorship Deals – youngandprofiting.com/sponsorships
Leave a Review – ratethispodcast.com/yap
Watch Videos – youtube.com/c/YoungandProfiting
Follow Hala Taha
LinkedIn – linkedin.com/in/htaha/
Instagram – instagram.com/yapwithhala/
TikTok – tiktok.com/@yapwithhala
Twitter – twitter.com/yapwithhala
Learn more about YAP Media’s Services – yapmedia.io/
[00:00:00] Hala Taha: Yeah, fam, welcome back to the show. This year, I've probably released about half a dozen AI episodes. And today, I'm releasing another AI episode. And this time, it's going to be about human centered AI. Now, human centered AI is sort of a new conversation that's happening in the AI world. In the past, it was all about what is AI?
How are these algorithms created? How are we enhancing these algorithms and tools? And now, AI is working really well, and the conversation has now shifted to human centered AI. How do we ensure that the AI has the utility that we want as humans? How do we make sure that it's safe? How do we make sure that AI is inclusive?
How do we make sure that AI is not going to take people's jobs? So now it's really focusing towards how do we use AI to optimize humanity? not how we're optimizing algorithms. I love this topic. I think it's super important. AI is a scary thing. A lot of us feel nervous and anxious about it. A lot of us feel excited about it.
And I can't wait to speak with Peter Norvig about this topic today. He is a fellow at Stanford's Human Centered AI Institute. He also formerly worked at Google and NASA. And he's literally written a textbook on AI. And he's been writing about the ethics of data science and AI since the 90s. So without further ado, here's my human centered AI conversation with Peter Norvig.
Peter, welcome to Young and Profiting Podcast.
[00:02:31] Peter Norvig: Great to be here. Thanks for having me.
[00:02:33] Hala Taha: I'm really looking forward to this conversation. I love talking about AI and I can't wait to pick your brain on that topic. But first, I want to talk a little bit about your career journey. I learned that you worked at some awesome companies like NASA.
You actually worked at Google, but it turns out you started in academia. So I'm curious to understand, why did you decide to transition from academia to the corporate world?
[00:02:58] Peter Norvig: So I've been in a lot of places. I'm a AI hipster. I was doing it before it was cool. Got interested in it as a subject in the 1980s.
And at that time, really the only way to pursue it was through academics. So got my PhD and it was the assumption back then that you get a PhD, you're going to go be a professor. There was much less back and forth between academics and industry than there is today. So that's the path I took. But then I started to realize.
We didn't quite have the word big data back then, but I saw that that's the way things were going. And I saw as a young assistant professor, I couldn't get the resources I needed. You could write a grant proposal, get a little bit of money, get a couple of computers and a couple of grad students, but I really couldn't get the resources to do the kind of big projects I wanted to do.
And industry was the only way to do that. So I set out on that path.
[00:03:57] Hala Taha: I love that. It's so funny that you say you were doing AI before people knew it was a thing. For me, it was surprising because I feel like we hear about AI so much, but it turns out that AI has been a thing for decades. Can you talk to us about when you first discovered AI and how long ago that was?
[00:04:14] Peter Norvig: So it's definitely been here right from the start. Alan Turing, one of the founders of the field, writing about it in 1956, foreseeing the chatbots that we have today. But of course, we didn't know how to build them back then. It was definitely part of the vision of where we might go. So I guess I got interested.
I was lucky. That I had a high school that at that time had a computer class and also had a class in linguistics and I took those two classes and talk to the teachers in the classes and say, hey, it seems like there's some overlap between those two. Can we get computers to understand English? And they said, yeah, that's a great subject, but we can't really teach you that that's beyond what we know how to do
so. You're on your own pursuing that goal and that's more or less what I've been doing since. With some side trips along the way.
[00:05:08] Hala Taha: I always say that skills are never lost, they're really just transferred. So I'm curious to understand, what skills do you feel like were an advantage for you in the corporate world that you took from academia?
[00:05:20] Peter Norvig: I certainly agree with that idea of transfer. I guess the idea of being able to tackle a complex problem, being able to move into an area that hadn't been done before. Academia is all about invention of the new, and for industry, it's a mix of, you want to make successful products, but sometimes in order to do that, you've got to invent something new, and that's harder to do.
You don't know what the demand for it is going to be. There's nothing to compare to. And yet you have to design a path to say, uh, we're going to go ahead and build this and we're going to put it out and customers are going to have to get used to it because it's not going to be familiar to them.
[00:06:05] Hala Taha: And speaking of building something new, you were responsible for Google search, and that was a while back when Google really was not starting off, but there was only 200 employees when you joined them in 2001.
So what was it like working for Google back then?
[00:06:20] Peter Norvig: So it was an awesome time. The company was three years old, 200 people all in one building. I came in. And I got the honor of getting to lead the search team for a while for about 5 years. So it's not like I invented it. Google search was already there, but they were 3 years old and it was really the time when they're trying to ramp up the advertising business.
So a lot of the key people who had built the search team and moved over to help build. The advertising platform and so there was an opening and I was had just come on board and so I got the opportunity to be a leader of the search team. And bring that forward over the next five years. So that was super exciting to be right in the middle of a transformative time in our industry.
[00:07:09] Hala Taha: Yeah. And I think a lot of my listeners, they don't realize that the internet was actually much different before Google. Google really changed the way that we use the internet. Can you help people understand what it was like before Google search?
[00:07:23] Peter Norvig: I guess there was a couple of things. First of all, there was.
Directories and lists of sites. I remember from the various early days, 1993 or so, and there was a site that was internet site of the day. And so it was just, you go there and it says, Hey, look, here's a new website that you might not have heard of before. And it was like, wow, you know, today, Ten new websites joined the web and they picked out a good one and you could keep up that way.
But then a year or two later, that no longer work because there were thousands of new sites every day, not just a couple. Yahoo was one of the first to try to deal with that. And they took this, you know, it's not going to be just one person saying, here's my favorite site today. It's going to be a company organizing the sites into directory structure.
And that worked okay. When the web was a little bit bigger, but as it continued to grow, that no longer worked. And then we really needed search rather than manually curated lists of directories and so on. But in the early days, the search systems just weren't that good. We had some experience as a field of doing, it used to be called information retrieval rather than search.
And it was sort of, it worked. The techniques we had at the time worked for things like libraries. But the problem there was, in a library, everything that was published is a real book, or a real journal article that's already been vetted. And so the quality is all at a pretty high level. On the web, that just wasn't true.
And so we needed new systems that not only said what's relevant to your query, but also What's the quality of this content and other companies really hadn't done that. And Google said, we're going to take this really seriously and we're going to work as hard as we can to solve that problem. And I think others didn't really see that as an opportunity.
So there's a story of in the very early days, people were saying, here's Google, it's rising. Yahoo was far bigger and far better known. Maybe Yahoo should buy Google. And that never happened in part because the Google founders. Thought they had something more important. Whereas Yahoo said, Oh, yeah, search.
That's kind of important We've got a home page and it's got all this stuff on it and you got to have search on the home page But you also need daily comics and horoscope. So why would search be more important than horoscope? You know, that's sort of how they felt about it and Google felt No, we think search is really really important and we're gonna do an excellent job of it So that was something new that other people hadn't thought about.
[00:10:05] Hala Taha: Totally. And people who are my age and all these listeners who are tuning in, Google is a verb for us. Google is how we use the internet. Something is changing now with AI. Now, a lot of us, instead of going to Google, we're going to chat GBT. And instead of putting in a search query and then digging around for information ourselves, We're just asking a question and getting Chachi Bouti to spit out the information.
So how do you think AI is going to change search and the way that we use the internet?
[00:10:37] Peter Norvig: I think there's always been changes and that's always been true. So Google's had a dominant position, but there's always lots of places that people go to. If you wanted breaking news, you went to Twitter. If you wanted a short explanation of something, you might go to Tik TOK or YouTube to see a video.
So, so it's going to be lots of ways to access this and we'll see how that changes. As AI gets better right now, sometimes it works and sometimes it doesn't, so it's a little bit of a frustrating experience. There certainly seems to be a path to say, we can have something that's a much better guide to what's out there, both in terms of answering a question immediately is one aspect, rather than saying, I'm going to be pointed to a site that has an answer.
I can get the answer right away. And then also guiding you through and maybe summarizing or giving you a whole. Learning path. So right now you sort of have to make up that path yourself, but I think I can do a good job of saying. Where are you now? What do you know? What do you want to know? And we're going to lead you through that.
[00:11:43] Hala Taha: Yeah. And AI also is just using the information that was inputted into the system, right? So it might not have all the information available that you could potentially find on the internet. Is that right?
[00:11:54] Peter Norvig: That's certainly true. Right. Depends on what it's trained on. And we're at a point right now where the training of these big AI models is very expensive.
So it's harder to keep them up to date. With the internet search, if something new happens, some new news is there. It's pretty fast of getting that index to making it available, but with the large AI models. It's just too expensive to update them instantaneously. And so you miss out on the newest stuff.
But that will change over time and we come up with new ways of getting things out faster and faster. When I first started at Google, we said we're kind of like a library where you can go to look things up. So it's okay that the library catalog only gets updated once a month. And now that would seem crazy to say you're only getting information that's a month old.
But in the earliest days of Google, that was the case. And then we went to daily and then hourly, and then even hourly wasn't fast enough and you had to get faster and faster.
[00:12:55] Hala Taha: Yeah, it's so interesting how fast technology changes. I know that you wrote a book about AI with Stuart Russell in 1995, you wrote a textbook, the first edition of Artificial Intelligence.
How has AI changed since you wrote that textbook?
[00:13:13] Peter Norvig: So we did the first edition in 95, and we're up to the fourth edition, which we did a year or two ago, and there definitely are changes. And first of all, I think we did the book because we saw changes even back in 1995, where in the earlier days, in the 80s and the start of the 90s, the Dominant form of AI was called expert system and what that meant was you build a system by going out and interviewing an expert, say an expert doctor and ask them in this situation with this patient, what would you do?
And then you try to build a system that would duplicate what the doctor said, and it was all built by hand programmers sitting down, trying to understand what the doctor said and trying to encode that. Into rules that they would write into the system, and it worked to some extent, but it was very brittle, and it just often failed to handle problems that were just slightly outside of what it had anticipated.
So, in the 1990s, there was a big switch away from this expert system, hand coded approach towards machine learning approaches where we said, rather than telling the system how to do it, you just show it lots of examples and let it learn by itself. And so we felt like the existing books had missed that change.
We wanted to write a book about it. So we did that. But of course, things continue to change. And so I guess, what can I say about what's changed over the four editions? I guess one was at the start, we felt like Well, AI, this is part of computer science and computer science is about algorithms. So we're going to show you a bunch of cool algorithms.
And we did that. And then in the second edition, I think we felt more like, okay, you still got to know all the cool algorithms, but if you had a choice, you're probably better off getting better data rather than getting better algorithms. So we're going to focus a lot more on what the data is. And that continued to be.
More true in the third edition. And now I feel like we've got plenty of data. We've got plenty of algorithms. You still have to know about them, but really the key to future progress is neither of those. The key is deciding what is it that you want? What is it that you're trying to build? So we have a great system that says, if you give me a bunch of data, I've got an algorithm that can optimize some objective that you're shooting for, but you got to tell me what the objective is.
What is it that you're trying to do? And for some tasks, that's easy. You know, if I'm playing chess, it's better to win than to lose. But in other tasks, that's the whole problem. And so we look at things like, we have these systems that help judges make decisions for parole, who gets out on parole and who doesn't.
And you want to parole somebody if they're going to behave well, and you want to not parole them if you think they're going to recommit a crime. But of course, these systems aren't going to be perfect. They're going to make mistakes. So the question you have to answer is what's the trade off between those mistakes?
How many innocent people should we jail to prevent one guilty person getting away, right? And so there's this trade off you're going to make. False positives and false negatives and what's one worth against another and even before there was AI or any kind of automation, we've had these kinds of discussions in our societies going back to Judge Blackman in England more than a century ago, who said it's better that ten guilty men go free than that one innocent man.
Be jailed now, I don't think he meant it that literally tends the boundary and nine's. Okay. And 11 would be bad, but with today's AI systems, you have to specify that, right? So you have to build the system and there's got to be an exact number in there of saying. What is the trade off point? And we're not very good at understanding how to do that.
We built a software industry and we have 50 years of experience in building debugging tools and so on, so we're pretty good at making reliable software. You know, every week you'll see some kind of bug or something, but we're getting pretty good at that. But we don't have a history of tools for saying how do we specify the right objective?
What are the trade offs? How important is it to avoid this mistake versus that mistake? And so we're kind of going by the seat of our pants and trying to figure that out. And so I think that's where a lot of the focus is now, is how do you decide what you really want?
I want to dig into this a bit because I think it ties in with this idea or the fact that AI is not yet, in all instances, at human level intelligence, and that's not always the goal.
[00:18:06] Hala Taha: I read some of your work where you said, Human level intelligence is really not always the goal when it comes to AI. So I want to read you a quote from Dr. Fei Fei Li, who came on the podcast episode 285. She's the co director of the Human Centered AI Institute, which you're also a fellow. And it was an awesome conversation.
And she said, the most advanced computer AI algorithm will still play a good chess move when the room is on fire. So she's trying to explain that AI doesn't have human level common sense. It's still going to play a chess move even when the room is on fire. So, let's start here. How do you feel AI stacks up right now against the human brain as a tool?
[00:18:47] Peter Norvig: So that's great. Fei Fei is awesome. I've heard many of her talks where she makes great points like that. I guess I would try to avoid trying to make metrics that are one dimensional.
[00:18:58] Hala Taha: Mm
[00:18:58] Peter Norvig: How does AI compare to humans? For a couple reasons. I don't want to say the purpose of A. I. is to replace humans.
We already know how to make human intelligence. My wife and I did it twice the old fashioned way. It worked out great. So instead of saying, can we make an A. I. that replaces a human, we should say, What kind of tools can we make so that humans and machines together will be more powerful? What's the right tool?
So we don't want a tool that replaces a human. We want a tool that fills in the missing pieces. And we've always had that. There's always been a mix of Subhuman and superhuman performance. So my calculator is much better at me at dividing 10 digit integers. So I rely on it rather than trying to work it out myself.
And I think we'll see more of that, of saying what are the right tools for people to use. Now, in terms of this, General AI versus narrow AI. I think that's really important. So there's multiple dimensions we want to measure. So we want to focus on both generality and performance. So how good are these machines and how general are they?
So, yes, we have fantastic chess playing programs that are Better than the best human chess players. And recently it's also true in go and we see sort of every week it's true. It's something else, but we haven't done quite as well at making them good at being general. So we have these large language models, the chat GPT and Gemini and so on, and they're good at being general.
They're not completely competent yet at doing that. So. They'll surprise you in both ways, so give you an amazingly good answer one time. And then the next time they'll give you an amazingly bad answer. So they're not reliable yet at being general. And then we have incredible tools that are narrow. And so we're kind of looking at this frontier of how can we make things.
Both perform better and more general. So I think we'll get to the point where we'll say here's an AI and it can make a chess move and it can also operate in the world. But right now we separate those two things out and we say we're going to have the chess program that only plays chess and then we're going to have the large language models and it won't be as good at chess, but it will be good at some aspects of figuring out what to do in unusual situations.
[00:21:29] Hala Taha: Could you give us some concrete examples of AI that we might want superior human level intelligence versus AI that we wouldn't want to have human level intelligence with?
[00:21:42] Peter Norvig: It's always better for it to be better, but sometimes we need that. And sometimes we don't, sometimes we want to make our own decisions.
And I guess part of that is I see too much of people saying, AI is going to be one dimensional and automation is going to be one dimensional and the more, the better. And I think that's the mistake that I'm worried about. And there's a great diagram from the society of automotive engineers, uh, Level of self driving cars, and they define that as five levels of self driving, and they did a great job of that.
And that's really useful. And now you can say, where is Waymo or Tesla? Are they at level 2 or level 3? Or what level are they at? And that was useful, but the diagram they used to accompany those levels was worrying to me. Because they've got this diagram, and at level 1, they have this icon of a person behind the car holding on to the steering wheel.
And then when you get up to level 5, that person has disappeared and they've just become a dot like outline. And so it's like, I don't want technology that makes me disappear. I want technology that respects me. And I don't want this trade off to be one dimensional of, if I get more automation, then I disappear more.
I'd rather have it be two dimensional and let me choose. So sometimes I might want to say, I've got a self driving car and I trust it. I just want to go to sleep. It should take over completely. But sometimes I might want to say, it can do all the hard parts, but I still want to be in control. I want to be able to say, oh, let's turn down that street, or go faster, or go slower, or let's make an unscheduled stop.
So I don't want to say just because I have automation that I've given up control. I want. Me to come first and let me make the choice of how much the machine is going to be doing and how much I'm going to keep control.
[00:23:37] Hala Taha: That makes a lot of sense. So like Dr. Lee, you are an advocate for human centered AI.
Can you help us understand what that is?
[00:23:45] Peter Norvig: I'm essentially a software engineer or programmer at heart. And so I look at what are the definitions of these various things and Software engineering is building systems that do the right thing. Artificial intelligence is also building systems that do the right thing.
So what's the difference? And I think the difference is that the enemy in software engineering is complexity. We have these programs with millions of lines. We have to get them right. And the enemy in AI is uncertainty. We don't know what the right answer is. And then, in human centered AI, the goal is to build systems that do the right thing for everyone, and do that fairly.
So that changes how you build these systems. And part of it is saying, you want to consider everybody involved. So you want to consider the users of your system, but you also want to consider the stakeholders and the effect on society as a whole. So we go back to, I was talking about this aid for judges and deciding who gets parole.
If you took a normal software engineering approach, you'd say, well, who's the user? Okay, it's this judge. So I want to make this program be great for them. A pretty display with graphs and charts and so on and numbers and figures and diagrams so that they can understand everything about the case and make a good decision.
And yes, you want that in human centered AI, but human centered AI says, we also got to consider the other stakeholders. So what's the effect on the defendant and their family? What's the effect on past victims and potential future victims and their family? What's the effect on society as a whole of mass incarceration or discrimination of various kinds?
So you're not just serving one user, you're serving all these different constituencies. I mentioned this idea of varying autonomy and control. So not having to give up control if you have more automation. And I think there's the aspect that it's multidisciplinary and multicultural. And I think too often you see companies say, okay, I want to build a system.
So the engineers will build it and get it working. And then afterwards we'll tack on this extra stuff to make it look better or make it more fair or less biased and so on. Yeah. And I think when you do that, you don't end up with good results. You've got to really bring in all these people right from the start, both in terms of being aware of what it means to build a system like this.
And then also that, as we were saying before, a lot of these problems is deciding what is it that we want? What is it that we're trying to optimize? And different people have different opinions on that. And so if you get a. Homogeneous group of engineers, they might all think the same thing and they say, great, we're agreed.
We must have the right answer. But then you go a little bit broader to other people from other parts of society, and they might say, no, you forgot about this other aspect. You're trying to optimize this one thing, but that doesn't work for us. So you've got to bring those people in right from the start to understand who all your potential users are and what's fair for all of them.
[00:27:00] Hala Taha: So one of the things that worries me is that we live in a capitalistic world. So while it's nice to think that people are going to have a human centered approach with AI, I do feel like at the end of the day, companies are going to do whatever's going to impact their bottom line most positively. So what are the ways that you think that There'll be some guardrails against not using AI in a human centered way.
[00:27:25] Peter Norvig: So that's certainly an issue with capitalism, not specifically for AI at all, right? That's across the board. So what do we have to combat that? Part of it is regulations of various kinds, so governments can set in and get rules. Part of that is. Pressure from the customers saying, here's the kind of company we want, here's the kind of products we want.
And part of that would be competition of saying, you build a system that doesn't respect something that users want. Somebody else will build 1 that's better. And I think we're in this kind of wild West period now, where we don't quite know what the bounds are going to be. There's so many of these sets of AI principles.
Now, all the big companies have their own sets. I helped put together the Google one, various countries have legislation or sets of principles. The white house put out their set of AI principles a couple of months ago. The professional societies, like the. Association of Computing Machinery has theirs. I actually joined an AI principles board with Underwriters Laboratory.
And I thought that was interesting because the last time, more than 100 years ago, there was a technology and people were worried that it was going to kill everyone. And it was electricity. Right. And so underwriters laboratory stepped in and said, okay, y'all are worried about getting electrocuted, but we're going to pick this little UL sticker on your toaster.
And that means you're probably not going to die and consumers trusted that mark. And therefore, the companies voluntarily submitted themselves to certification and I kind of feel like this. Third party non profit certification can be more agile than government making laws. And so I think that's part of the solution.
But I don't think any one part of it can do it all by himself. I think we need all those parts.
[00:29:36] Hala Taha: Yeah, very cool. Very interesting. I agree. A third party solution sounds like it could work pretty well. So we had Sal Khan on the show and he, as the Khan Academy, he talked a lot about how AI could help education.
Do you have any ideas of how AI could support education and students?
[00:29:55] Peter Norvig: Yeah, I think that's awesome. I think the work Sal is doing has been great right from the start and recently over the last year or so with the Conmigo large language model. So, back in 2011, Sebastian Thrun and I said, we want to take advantage of this capability for online education.
And we put together. An online course about a I signed up 100, 000 students far more than we ever expected to sign up and we ran that course. But, of course, at that time, the leading technology was YouTube show students a video and then we'd have them answer a question and we could do a little bit. You know, if they got.
If they got this wrong answer, we could show them one thing, and if they got another wrong answer, we could show them something else. But basically, it was very limited in the flow you could do. And now, with these large language models, you have a much better chance to customize the results for the student, both in terms of the learning experience, and then I think also in terms of the motivation for the student.
So that was the one thing we learned in doing the class, is that, We came in saying, well, our job is really information. If we can explain things clearly, then we're done, and we're a success. And we soon realized that that's only part of the job, and really the motivation is more important than the information.
Because if a student drops out, it doesn't matter how good our explanations are, if they're not watching them anymore. It doesn't do any good. And so I think AI has this capability to motivate much better, to allow students to do what they're interested in, rather than what the teacher says they should be interested in.
But we got a ways to go yet, and we don't quite know how to do that, right? So you can't just plug in a language model and hope that it's going to work. So yes, it would be useful. You have to train it to be a teacher as well as to understand what it's talking about. And we haven't quite done that yet.
We're on the way to doing that. There's a dozen different problems to be solved, and we have candidate solutions, but we haven't done it all. So right now, the language models can be badgered too easily. You say, here's a problem, and the student says, tell me the answer. And at first, the language model would say, no, you wouldn't learn anything if I told you the answer.
But then you say, tell me the answer, please. And it says, oh, okay. And so we have to teach these things. When is it? the right thing to give the student the answer? When is it the right thing to be tough and refuse to do that? When should you say, Oh, you're right. That's a hard problem. Here's a simpler problem.
Why don't you try the simpler problem first? Or to say, it looks like you're getting frustrated. Why don't we take a break? Or why don't we go back and do something else that would be more fun for you? And so there's all these moves. That teachers can take and so doing education. Well, is this combination of really knowing the subject matter and then really knowing the student and the pedagogical moves you can make and we haven't quite yet built a system that's an expert on both of those, but con and others are working on it.
And so I think it's a great and exciting opportunity.
[00:33:18] Hala Taha: Do you feel like some of this learning and training could be applied to the workplace?
[00:33:23] Peter Norvig: Yeah, absolutely. And some of it, I think, is easier and better done for workplace training. And I think that's going to be really important. We've built this bizarre system now where we say, You should go to a college for four years, and then we're going to hand you a piece of paper that says you never have to learn anything again.
That shouldn't be the way we do things. And there's a value to college. Maybe it doesn't have to be for everybody. Maybe more people could be learning more on the job, or learning just in time when they need a new skill. So I think there's a great opportunity for that. I think that the systems we have right now are better at shorter subjects anyways.
So it's hard to put together a class that says, let's do all of biology one or something. But it's easier to say, why don't you get trained on this specific. Workplace thing, how to operate this machine, or how to operate the software and so on. So, in some sense, we're better at that kind of training than we are at the traditional schooling.
So, yeah, there's definitely a big opportunity there. The thing that mitigates against it is we could spend a lot of. investment on making the perfect biology one class, because there's going to be millions of students that take it for some of this on the job training. You know, I'm in a small company and we do things a specific way and there might be only five people.
That need to be trained on it. So right now it's not really cost effective to say, can I build a system that will do that training? But that's 1 of the goals to say, can we make it easier for somebody who's not an expert programmer, not an AI expert to say, here's some topic I want to teach and I should be able to go ahead and teach that.
And I think that's something that's oddly missing from our standard playbook. So you look at, we have these office suites, and what do they give you? They give you word processing and spreadsheets and PowerPoint presentations. And sure, that's great. Those are three things that I want. But I think a lot of people want this.
I want to be able to train somebody on a specific topic more than they want spreadsheets. But we don't have that yet. But maybe someday we will. Maybe that will be a standard tool that will be available to everyone. Thank you.
[00:35:46] Hala Taha: Sounds really cool. So this conversation made me realize that there really is no better time to be an entrepreneur.
Because as we were talking about, A lot of jobs might get replaced by AI. And when you're an entrepreneur, when you own the business, you're sort of in control of all those decisions. And you're the one who might end up benefiting from the cost savings of replacing a human with AI. So do you feel like AI is going to generate a lot more entrepreneurs and solopreneurs in the future?
[00:36:16] Peter Norvig: Absolutely. Absolutely. It's a combination, so I think AI is a big part of it. I think the internet and access to data was part of it. The cloud computing was a big part of it, right? So it used to be, if you were a software engineer, the hardest part was raising money because you had to buy a lot of computers.
Just to get started now, all you need is a laptops and a Starbucks card and you can sit there and start going and then rent out the cloud computing resources as you need them and pay as you go. And so I think I will have a similar type of effect of you can now start doing things. Much more quickly, you can prototype something, go to a release product much faster, and it'll also make it more widely available.
So I live in Silicon Valley, so I see all these notices going around of saying, looking for a technical co founder. So there's lots of people that say, well, I have an idea, but I'm not enough of a programmer to do it, so I need somebody else to help me do it. I think in the future, a lot of those people will be able to do it themselves.
So I had a great example of a friend who's a biologist and he said, I'm not a programmer. I can pull some data out of a spreadsheet and make a chart, but I can't do much more than that. But I study bird migrations and I always wanted to have this interactive map of where the birds are going and play with that.
And he said, and I knew a real programmer could do it, but it was way beyond me. But then I, I heard about this co pilot and I started playing around with it and I built the app by myself. So I think we'll see a lot more of that people that are non technical or semi technical who previously thought here's something that's way beyond what I could ever do.
I need to find somebody else to do it. Now I can do it myself.
[00:38:09] Hala Taha: Yeah. I totally agree. And we're seeing it first with the arts. For example, now you can use Dolly and be a graphic designer, you can use LGBT and be a writer. So so many of the marketing things are already being outsourced by AI. It's only a matter of time where some of these more difficult things like creating an app, like you were saying, is going to be able to be done with AI.
[00:38:31] Peter Norvig: Absolutely.
[00:38:33] Hala Taha: Cool. So what are the ways that you advise that entrepreneurs use AI in the workplace right now?
[00:38:37] Peter Norvig: You could help build prototype systems like that. You can do research. You can ask, give me a summary of this topic. What are the important things? What do I need to know? As you said, creating artwork and so on, if that's not a skill you have, they can definitely help you do that.
Looking for things that you don't know is useful. And so I think just being aware of what the possibilities are and having that as one of the things that you can call upon. It's not going to solve everything for you, but it just makes everything go a little bit faster.
[00:39:10] Hala Taha: Do you think that AI is going to help accelerate income inequality?
[00:39:15] Peter Norvig: Absolutely. Absolutely. I think it's kind of mixed any kind of software or any kind of goods with zero marginal cost tends to concentrate wealth in the hands of a few. And so that's definitely something to be worried about with AI. We also have this aspect that the very largest. Models are big and expensive.
They require big capital investments. And if you'd asked me two years ago, I would have said, Oh, all the A. I. Is going to migrate to the big cloud providers. Because they're going to be the only ones that can build these large state of the art models. But I think we're already going past that, right? So we're now seeing these much smaller open source models that are almost as good, and that don't impose a barrier of huge upfront cost.
So I think there's an opportunity. Yes, the big companies are going to get bigger because of this, but I think there's also this opportunity for the small opportunistic entrepreneur to say here's an opening and I can move much faster than I could before and I can build something and get it done and then have that available.
So that's part of it Then the other part is well, what about people who aren't? Entrepreneurs. And we've seen some encouraging research that says AI right now does alleviate inequality. And so there have been studies looking at, well, you bring AI assistance into Call center and it helps the less skilled people more than the more skilled people, which makes sense, right?
The people who are more skilled, they already know all the answers and the people that were less skilled. It brings them up almost to the same level. So, I think that's encouraging because that means there's going to be a lot of people who are able to upscale what they do and they'll get higher paying jobs.
They're not going to found their own company, but they're going to do better because they're going to have better skills.
[00:41:18] Hala Taha: Makes a lot of sense. Okay. So as we close out this interview, let's talk about the future a bit. What scares you the most about AI right now?
[00:41:28] Peter Norvig: I'm not worried about these Terminator scenarios of an AI waking up and saying I think I'll kill all humans today.
So what am I worried about? I guess I'm more worried about a human waking up and saying I want to do something bad today. So what could that be? Well, misinformation, we've seen a lot of that, and I think it's mixed, uh, how big an effect AI will have on that. I mean, it's already pretty easy to go out and hire somebody to create fake news and promulgate it.
And the hard part really is getting it to be popular, not to create it in the first place. So in some sense, maybe AI doesn't make that much difference. It's still just as hard to get it out. And maybe I can fight against that misinformation. So I think the jury is still out on that. But if you did get to the point where an AI knew enough about an individual user to say, I'm going to create the fake news, that's going to be effective specifically for you.
That would be really worrying and we're not there yet, but that's something to worry about. I worry about the future of. Warfare. So you're seeing these things today, we just saw a tiny little personal size drone shot down a Russian helicopter. So we've had half a century of or so of mostly a stalemate of saying the big countries have the power to impose themselves on the others, but none of them are really going to unilaterally do it on a large way.
And we have smaller regional conflicts. Now we may be transitioning into a world where we say the power is not just in the big countries, it's in lots of smaller groups. And that becomes a more volatile situation. And so there could be more of these smaller regional conflicts and more worries for civilians that get caught up in it.
So I'm worried about that as well. And then, like you said, the income inequality, I think is a big issue.
[00:43:28] Hala Taha: Well, let's end on a positive note. I guess what excites you the most about AI?
[00:43:35] Peter Norvig: So a big part of it is this opportunity for education. That's where I spent some of my time, and I'm really interested in that now.
So I think that can make things better for everyone. It's just making everyone more powerful, more able to do their job, able to get a better job. So that's exciting. I think. Applications in healthcare are a great opportunity, and I got involved a little bit in trying to have, uh, better digital health records, and that really didn't go so far, mostly because of bureaucracy and so on, but I think we have the opportunity now to do a much better job, to invent new treatments, And new drugs, you've seen things like AlphaFold figures out, here's how every protein works.
And, you know, it used to be you could get a PhD for figuring out how one protein worked. And AlphaFold said, I did them all. So I think this will lead to drug discovery, lead to healthier lives, longevity, and so on. So that's a really exciting application.
[00:44:37] Hala Taha: It's so interesting to me that AI can do so much good, and then there's also such a risk of it doing so much bad, but I feel like any good technology brings that risk along with it.
[00:44:49] Peter Norvig: I think that's always true, right? If it's a powerful technology, you can do good or bad, especially if there are good and bad people trying to harness that way. And some of it is intentional bad uses and some of it is unintentional. So, internal combustion engines did amazing things in terms of distributing food worldwide and Making that be available, making transportation be available, but there are also these unintended side effects of pollution and global warming and some bad effects on the structure of cities and so on.
And we would be a lot better off if when cars were first starting to roll out in 1900, if somebody said, let's think about these long term effects. So, I guess I'm optimistic that there are people now thinking about these effects for as we're just starting to roll it out. So. Maybe we'll have a better outcome.
[00:45:40] Hala Taha: Yeah, I hope so. Well, Peter, thank you so much for joining the show. I end my show with two questions that I ask all of my guests. What is one actionable thing our young improfiters can do today to become more profitable tomorrow?
[00:45:52] Peter Norvig: I Keep your eye on what it is that people want. So I said, the problem in AI is figuring out what we want.
I'd work some with people at Y Combinator, and I still have this t shirt that says on the back, make something people want. And very simple advice to entrepreneurs, but sometimes missed. And so I think that's true generally, and I think AI can help us do that.
[00:46:17] Hala Taha: Yeah, it's so true. The number one reason why entrepreneurs and startups fail is because there's no market demand.
So make something that people want. And what is your secret to profiting in life? And this can go beyond today's episode topic.
[00:46:32] Peter Norvig: Keep around the people you like and be kind to everybody.
[00:46:37] Hala Taha: Love that. Where can everybody learn more about you and everything that you do?
[00:46:42] Peter Norvig: You can look for me at Norvig. com or on LinkedIn or Thanks to Google, I'm easy to find.
[00:46:50] Hala Taha: Awesome. I'll stick all your links in the show notes, Peter. Thank you so much for joining us.
[00:46:54] Peter Norvig: Great to join you, Hala.
[00:47:00] Hala Taha: Well, young improfiters, I hope you learned something from my conversation with Peter. It's so fascinating to hear about the technology and its implications from someone who has been on the front lines of some huge transformations in how we live and work. Peter was, like he said, doing AI long before it was cool, and therefore has some interesting observations about its capabilities and where it's headed.
He says that rather than thinking about AI as something that We should be thinking about it as a tool that fills in the gaps and helps humans achieve greater things. This could even include providing assistance with school or on the job training, with AI serving as the ultimate personalized tutor or job trainer.
AI is also going to continue what the internet, cloud computing, and other advances have already started. It's going to make it easier and easier easier to launch your own business and become an entrepreneur. The number of entrepreneurs are going to keep growing and growing and being able to incorporate AI into your business.
Combining the human and the superhuman will help set you apart, but it's also important to remember that AI is a tool and like any tool it could be used for good or bad. It's up to us to shape the future of AI in a way that benefits everyone. Thanks for listening to this episode of Young and Profiting.
Every time you listen to and enjoy an episode of this podcast, share it with your friends or your family. Perhaps someday an AI bot can do this for you, but until then, we depend on you. And if you did enjoy this show and you learned something, then please take a couple minutes to drop us a five star review on Apple Podcasts, Spotify, or wherever you listen to your podcast.
Nothing helps us reach more people than a good review from our loyal listeners. If you prefer to watch your podcast as videos, you can find us on YouTube. Just look up Young and Profiting and you'll find all of our episodes on there. If you're looking for me, you can find me on Instagram at yapwithhala or LinkedIn by searching my name.
It's Hala Taha. Before we wrap up, I want to give a big shout out to my incredible Yap production team. Thank you so much for your hard work. This is your host Hala Taha, aka the podcast princess signing off.
Episode Transcription
Enter your name and email address below and I'll send you periodic updates about the podcast.