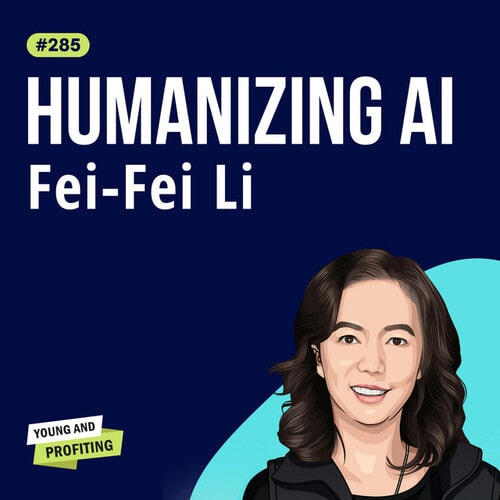
Fei-Fei Li: The “Godmother of AI”, Keeping Humanity at the Heart of the AI Revolution | E285
Fei-Fei Li: The “Godmother of AI”, Keeping Humanity at the Heart of the AI Revolution | E285
Dr. Fei-Fei Li is a professor of Computer Science at Stanford University and the co-director of the Stanford Institute for Human-Centered AI. She is the creator of ImageNet, a key driver of modern artificial intelligence. With over 20 years at the forefront of the field, Dr. Li is focused on AI research, education, and policy to improve the human condition.
In this episode, Hala and Fei-Fei will discuss:
– The current capabilities of AI
– The difference between machine learning and AI
– The training process for AI models
– The gaps in our knowledge about how AI learns
– Why ChatGPT fails at higher-level reasoning like math
– The biological inspiration for vision in computers
– Fears and hopes associated with AI
– The human element of jobs AI can’t replace
– Augmentation of human capabilities through AI
– The three pillars of her human-centered AI framework
– Responsible development and use of AI
– The roadblocks to be aware of when using AI
– Her advice to young entrepreneurs navigating the AI world
– And other topics…
Dr. Fei-Fei Li is a professor of Computer Science at Stanford University and the co-director of the Stanford Institute for Human-Centered AI. She is also the creator of ImageNet and the ImageNet Challenge, a key catalyst to the latest developments in deep learning and AI. Sometimes called the ‘Godmother of AI,’ she is a pioneer in early computer vision research. Dr. Li is the author of The Worlds I See, one of Barack Obama’s recommended books on AI. Her work has been featured in various publications, including the New York Times, Wall Street Journal, Fortune Magazine, Science, and Wired Magazine.
Connect with Fei-Fei:
Fei-Fei’s Bio: https://profiles.stanford.edu/fei-fei-li
Fei-Fei’s LinkedIn: https://www.linkedin.com/in/fei-fei-li-4541247/
Fei-Fei’s Twitter: https://twitter.com/drfeifei
Resources Mentioned:
Fei-Fei’s Book, The Worlds I See: Curiosity, Exploration, and Discovery at the Dawn of AI:
Stanford Human Center AI Institute Website: https://hai.stanford.edu/
LinkedIn Secrets Masterclass, Have Job Security For Life:
Use code ‘podcast’ for 30% off at yapmedia.io/course
Sponsored By:
Shopify – Sign up for a one-dollar-per-month trial period at youngandprofiting.co/shopify
Indeed – Get a $75 job credit at indeed.com/profiting
Yahoo Finance – For comprehensive financial news and analysis, visit YahooFinance.com
More About Young and Profiting
Download Transcripts – youngandprofiting.com
Get Sponsorship Deals – youngandprofiting.com/sponsorships
Leave a Review – ratethispodcast.com/yap
Watch Videos – youtube.com/c/YoungandProfiting
Follow Hala Taha
LinkedIn – linkedin.com/in/htaha/
Instagram – instagram.com/yapwithhala/
TikTok – tiktok.com/@yapwithhala
Twitter – twitter.com/yapwithhala
Learn more about YAP Media’s Services – yapmedia.io/
[00:00:00] Hala Taha: yeah, fam, welcome to the show and I'm super pumped to be digging even deeper today into the field of artificial intelligence and how it might impact all of our lives in the years to come. Today, we have a special guest who has played a big role in the development of AI and will likely even play a bigger role in how it gets used in the future.
Dr. Fei Fei Li is a professor of computer science at Stanford University, as well as the co director of the Stanford Institute for Human Centered AI. Her work focuses on advancing AI research, education, and policy to improve the human condition. Dr. Li's new book is called The World's I See, and it weaves together her personal narrative with the history and development of AI.
Today we're going to talk about her human centered approach to AI, we're going to discuss how she's creating eyes for AI with computer visioning, and we'll also learn what the future holds for the promising, yet sometimes scary, technology of AI. Dr. Lee, welcome to Young and Profiting Podcast
[00:02:36] Fei-Fei Li: Thank you, Hala. I'm very excited to join the show.
[00:02:40] Hala Taha: Likewise, I'm so honored to talk to somebody like you, given all your credentials. In fact, Wired named you one of a tiny group of scientists, perhaps small enough to fit around a kitchen table, to Who's responsible for AI's recent remarkable advances.
So it feels like AI is changing every day. There's new developments all the time. So my first question to you is, can you walk us through the development of AI? What can it currently do now? And what can't it do right now?
[00:03:08] Fei-Fei Li: Yeah, great question. It's true. Even as an AI scientist, I feel that I can hardly catch up with the progress of AI, right?
It is. a young field of around 70 years old, but it's progressing really, really fast. So what can I do right now? First of all, it's already everywhere. It's around us. Another name for AI that is a little less of a hype name is machine learning. It's really just mathematical models built by computer programs so that the program can iterate and learn to make the model.
Predict or decide on data better. So it's fundamentally machine learning. For example, if we shop on Amazon app, the kind of recommendations we get is through machine learning or A. I. If you go from place A to place B, the algorithm that gets you the road to map out the path is machine learning. If you, um, Go to Netflix.
There is a recommendation that's machine learning. If you watch a movie, there is a lot of machine learning, computer vision, computer graphics, to make special effects, to make animations. That's machine learning. So machine learning and AI is already everywhere. What cannot do? Well, no machines today can help me to fold my laundry or cook my omelet.
It cannot take away complex human reasoning, it cannot create in the way humans create in the combination of both reasoning, logic, but also beauty, emotion, There's a quote from 1970s about AI, and I think that quote still is true today. It says, the most advanced computer AI algorithm will still play a good chess move when the room is on fire.
It's a quote to show that machines are programmed to do tasks. But it's unlike humans. We have a much more fluid, organic, contextual, situational awareness of And that's what our own thinking, our own emotion, as well as the surrounding. And that is not what AI is today.
[00:05:32] Hala Taha: So insightful. And I love that you said that it's like an evolution of machine learning, because I always wonder, well, what's the difference between machine learning and AI?
It sounds pretty similar. So machine learning was almost like the basics of AI.
[00:05:47] Fei-Fei Li: The tool of AI. Think about physics. Physics in Newtonian time, the most important tool of physics was calculus. Yet, we call the field physics. So artificial intelligence is a scientific field that is researching and developing technology to make machines think like humans.
But the tools we use, the mathematical computer science tool, is dominated by machine learning, especially neural network algorithms. So good.
[00:06:19] Hala Taha: So AI is actually fresh on my mind because two days ago I interviewed Dr. Stephen Wolfram, um, And we talked about ChatGBT and how ChatGBT works. And he was explaining to me that when they were developing ChatGBT, what was surprising is that they found out that these simple rules.
Would create all this complexity that they could give Chachi Bouti simple rules, and then it could write like a human. And it turns out that we actually still don't really understand how AI learns, which to me is like mind boggling. How did we create something? And yet we don't even know how it really works.
Can you elaborate on that a bit?
[00:06:57] Fei-Fei Li: Really at the end of the day. There are things we understand, there are things we don't. So it's neither a white box nor a black box. I would call it a gray box. And depending on your understanding of the AI technology, it's either darker gray or lighter gray. So the things we know is that It is a neural network algorithm that is behind, say, a chat GPT model or a large language model.
Of course, you hear the names of transformer models, sequence to sequence and all that. At the end of the day, these models take data, like document data, And it learns how the words, and sometimes even sub words, right, parts of words, are connected with each other, there are patterns to see, right? If you see the word, how, it tends to be followed by R, and then it tends to be followed by U, so how are you is a frequently occurring sequence, so that pattern is learned.
And once you learn enough in a big, huge neural network, your ability to predict the next word when you're given a word is really, really quite amazing. Amazingly high to the point that It can converse more or less like a human, and because in the training data it has so much knowledge, whether it's chemistry or movie reviews or geopolitical facts, it has memorized all of them, so it can give out very, very good answers.
So those are the things we know. We know how the algorithm works. We know it needs training. We know that it's learning and predicting pattern. What we don't know is that Because these models are huge, there are billions and billions, hundreds of billions of parameters. And then inside these models, there are these.
Little nodes, each one of them have a little mathematical function that connects to each other. So how do we know exactly how these billions and billions of parameters learn the pattern and where is the pattern stored? And why sometimes it hallucinates a pattern versus it gives out a correct answer.
There is not yet precise mathematical explanation. We don't know, there's no equation that can tell us, Oh, I know exactly why at this moment. The chat GPT gives you the word, how are you versus how is he, you know, so that's where the grayness come from. These are large models with behaviors that are not precisely explained mathematically.
[00:09:56] Hala Taha: talk to us about how AI models are trained. How does AI learn typically? Typically
[00:10:03] Fei-Fei Li: AI model is given a vast amount of data. And then some of the data are labeled with human supervision. Like if I give AI models millions and millions of images, some are labeled cats, dogs, microwaves, chairs, and all that.
And they learn to associate the pattern with the labels. Sometimes, in recent, especially in language domain, what we call self supervision, you give it millions and millions, trillions of documents, and it just keeps learning to predict the next syllabus, the next word, because all the training data is showing you all these, uh, sequences of words.
And there, you don't have to give additional label. You just give the documents and that's called self supervised learning. So whether it's supervised with additional labels or supervised. Without additional label is self supervised, it starts with data. Now, data goes into the algorithm, and the algorithm has to have an objective to learn.
Typically, in the language model, the objective is to predict the next syllabus as accurately as the training data shows you. In the case of images with cat labels, for example, is to predict the an image that has a cat with the right label cat instead of the wrong label microwave. And then because it has this objective during training, if it makes a mistake, if I didn't predict the next word right, or if I labeled the cat wrong, it goes back and iterates and updates its parameters based on the mistake.
It has some mathematical rules. Or learning rules to update and then they just keeps doing that till it humans ask it to stop or it no longer updates whatever stop criteria and then you're left with a ginormous neural network that's already trained by ginormous amount of data and in that neural network, it has all the parameters, the mathematical parameters that's already learned now, uh, You can take this, and now you have a new sentence come in.
And then it goes through this model, because it has all the parameters it has learned, it predicts what I should say, given the new sentence. Like, Hello Hala, how is your breakfast today? And it would predict, I had a great breakfast today, or whatever. So that's how it's done.
[00:12:45] Hala Taha: It's just predicting the next word and the next word and the next word based on all the different patterns and trying to figure out what makes sense to come next. So that's super clear. What I don't understand with something like Chachiwiti is that it's so good at writing human language, but it's known to make simple math mistakes.
How is it possible that it's good at doing human language, but then on math, for example, it's known to make stupid mistakes?
[00:13:11] Fei-Fei Li: It's because math, the way we do math in human mind is different from the way we do language. Language has a very clear pattern of sequence to sequence, like I say the word how the word are and you typically follow, but sometimes it doesn't, right?
So I have to learn these patterns. But if I say the word one plus. It's not like five typically follows or two typically follows, right? Like, there is actually a deeper rule of one plus two equals three. Of course, when it has seen enough of that, it should predict three for today's language model. And actually, it does.
This is too simple an example. But the point is that math takes a higher level of reasoning than just following statistical patterns. A large language model, by and large, follows statistical patterns. So some of the mathematical reasoning is still Lacking.
[00:14:11] Hala Taha: Totally makes sense. So you've got a new book.
It's called The Worlds I See. And you say that the worlds you see are in different dimensions. So can you talk to us about why you titled the book this way?
[00:14:23] Fei-Fei Li: Yeah. This title came about after I finished writing the book. And I realized the journey of writing the book is really peeling into different experiences.
Yeah. Totally. Totally. There is the world of AI that I experienced as a scientist. The book is a coming of age of a young scientist. So I experienced the world of science in different stages. But there is also the world as an immigrant. I go through life in different parts of the world, and how do I handle or go through that?
And then there is more subtle but profound world like learning to be a human. I know this sounds silly, but especially in the context of an AI scientist, it's really important. And part of the book is exploring my journey of learning. Living and taking care of ailing parents and how that experience build my own character, how we help each other, support each other.
And towards the end of the book, how that experience made me see my science in a different light compared to maybe other scientists who haven't had this very profound human experience. So it really is different worlds that I experienced and it's. blended into the book.
[00:15:47] Hala Taha: I love that. And I love how you call it a science memoir.
And so you say that you're involved in the science of AI, but you're also involved in the social aspect of AI. So what do you mean by the social aspect exactly?
[00:16:00] Fei-Fei Li: I started in AI as a very personal journey. It's just a young science nerd, loves an obscure niche, like nobody knows, field. But I'm just fascinated in a private way.
That how do we make machines think? How do we make machines see? And that, I was happy. And I would have been content with that through the rest of my life, honestly. Even if nobody in the world has heard of AI, I would be happily in my lab being a scientist. But what really changed is around 2017, 2018, I felt like me as a scientist and the tech world changed.
Woke up and realized, oh, wow, this technology has come to a maturation point that is impacting society. And because it's AI, it's inspired by human thinking, it's inspired by human behavior. It has so much human implication at the individual level as well as the society level. So as a scientist, I feel I was thrusted into a messier reality that I never really realized.
Now, I have a choice. A lot of my fellow scientists would just continue to stay in the lab, which I think it's very admirable and respected, still just focus on the science. But my other choice is to recognize as a scientist, as an educator, as a citizen, I have social responsibility. My responsibility is more focused on what I need to educate young people.
While I can teach them equations and coding and all that, I also want to share with them what the social implications are of this science because it's my responsibility. I also have a responsibility to communicate with the world because even starting quite a few years ago, now it's even worse because of the large language model.
There's just so much public discourse about AI and many of them are ill informed and that's dangerous. That's unfair, that's dangerous, it tends to harm people who are not in the position of power, and I have a responsibility to communicate. And then third, I also feel Stanford, especially as one of America's higher institutions, have a responsibility to help make the world better, to help our policymakers, to help civil society, to help companies, to help entrepreneurs.
To educate, to inform, and to give insights. And that is the messiness of meeting the real world, and I feel I shouldn't shy away from that. I should take on that responsibility.
[00:19:02] Hala Taha: Yeah, for sure. You're one of the most knowledgeable people about AI. We need you to tell us the roadblocks that we need to look out for, and how can we make sure that we use AI for good and not for bad, and take the steps to do that.
So let's talk about computer vision next. So you are a computer vision AI scientist. So what first got you interested in this and what is computer vision AI?
[00:19:26] Fei-Fei Li: Well, in one sentence, computer vision AI is part of AI is the specific part of AI that makes computers see and understand what it sees. And this is very profound.
When humans open our eyes. We see the world not only in colors and shades, we see it in meaning, right? Like I'm looking at my messy desk right now. It has cell phones. It has a cup. It has monitor, it has my allergy medicine, and it has a lot of meaning. And more than that, we can also construct. Even if we're not the best artists, humans since the beginning dawn of civilization, have been drawing about the world, has been sculpting about the world, has been building bridges and monuments, and has created the visual and the world.
So the ability to see and visually create and understand is so innate in humans. And wouldn't it be great if computers have that ability? And that is what computer vision is.
[00:20:36] Hala Taha: So interesting. When I think about consciousness, everything that has consciousness has eyes. This always like freaked me out. Bugs have eyes, fish have eyes,
Fish eyes look like our eyes. And that's so scary, weird. The fact that all these living things have eyes. If A. I. starts to have eyes, wouldn't it just be that they're living and sentient at that point?
[00:21:00] Fei-Fei Li: So first of all, Hala, you touched on something really, really profound, because visual sensing is one of the oldest, evolutionarily speaking.
So 540 million years ago, animals started developing eyes. It was a pinhole that collects light, but evolving to The kind of eyes, the fish, the octopus, the elephant, the eyes we have. So you actually touch on something really profound. This is extremely innate, embedded into our development of our intelligence.
And, uh, of course, you also ask a philosophically really profound question. Everything has eyes, has consciousness. Actually, a neuroscientist or neurophilosopher You should invite one to debate with you. For example, does a tiny shrimp using eyes doing things, does it have consciousness or it has just perception?
I don't have an answer, honestly. How do you measure consciousness, right? Just because the shrimp can see the rock and climb around, does it mean it's just a sensory reflex or it has a deeper consciousness? I don't know. So just because machines have eyes, does it develop consciousness? It's a topic we can talk about, but I just want to make sure that we are at least on the same page that just seeing itself doesn't mean it has consciousness.
But the kind of visual intelligence we have, like I just described, to understand, to create, to build, to represent a world with such visual complexity, at least in humans, it does take consciousness.
[00:22:50] Hala Taha: Everything that you're saying is just so interesting. Even that shrimp example, even though it's navigating, swimming around rocks and whatever, doesn't mean that it's actually conscious.
It could be, to your point, just all like reflexes. And that makes it a little less scary if machines end up having eyes. So how are you replicating biological processes like vision in computers now?
[00:23:12] Fei-Fei Li: I think a lot of computer vision is biologically inspired and it's inspiring in at least two areas. One is the algorithm itself.
So the whole neural network algorithm. In fact, back in the 1950s and 60s, the computer scientists were inspired by vision. Neuroscientists, when they were studying cat mammalian visual system, they discover the kind of hierarchical neurons, and it's because of that, it inspired computer scientists to build a neural network algorithm.
So the visual, the animal visual structure in the brain is very much the foundational inspiration to today's AI technology. So that's one area. The second inspiration come from functionality, right? The ability to see what do we see? Humans are not that good at seeing color. For example, we see color rich enough, but the truth is.
There's infinite wavelength that defines infinite colors, but we have only probably dozens of colors. So clearly we're not seeing just colors in the same way like if I use a machine to register wavelength. on the other hand, we see meaning, we see emotion, we see all these things. And it's just incredibly inspiring.
That we can, build these functionality into machines. And that is another part of biological inspiration. It's the functional inspiration. And with that, I think there is a lot to imagine. For example, first of all, visually impaired patients, if we help them with artificial visual system to understand the world, rich world we see, it will be tremendously helpful.
Machines, right? I don't know, do you have a Roomba in your house? Yeah. Yeah. Right. So it almost, it's kind of seeing, it's not seeing the same way we are, but it's kind of seeing a mapping. But one day I hope I not only have a Roomba, I also have a cleaning robot, right? Like then it needs to see my house in a much more complex way.
And then, uh, the most important, right, for example, rescue robots. There's so many situations that puts humans in danger. Or humans are already in danger and you want to rescue humans, but you don't want to put more humans in danger. Think about that Fukushima nuclear leak incident. People had to really sacrifice to go in there to stop the leak and all that.
It would be amazing if robots can do that. And that needs seeing. It needs visual intelligence in much deeper ways.
[00:25:58] Hala Taha: That's so interesting. And it's helpful for you to say that because my first reaction is like, why are we giving robots this much power? Like losing our power as humans. But to your point. It can help humans, and I know that's a whole, like, what you talk about is human centered AI, right?
Yes. Can you define what human centered AI is in your own words?
[00:26:19] Fei-Fei Li: Yeah, human centered AI is a framework of developing and using AI, and that framework puts humans, human values, into practice. human dignity in the center so that we're not developing technology that's harmful to humans. So it's really a way to see technology or use technology in a benevolent way.
Now, I'm not naive. I know technology is a double edged sword. I know that double edged sword can be used intentionally or unintentionally in bad ways. So human centered AI is really trying to underscore that we have a collective responsibility to focus on the good development and good use of AI. And it was really inspired by my time in industry when I was on sabbatical as a professor is seeing the incredible business opportunities.
That is already opening the flood gate of AI back in 2018. And knowing that when business start to use AI, it impacts lives of every individual. So I went back to Stanford and together with my colleagues, We realized as a thought leadership institution, as Americans higher education place to educate the next generation students, we should really have a point of view to develop and stay at the forefront of the development of this technology.
This is how we formulated the human centered AI framework.
[00:28:02] Hala Taha: And one of the biggest fears that people have with AI is that AI is going to replace all of our jobs. Now, AI is probably going to create a lot of jobs, and I've talked a lot about that with other guests on the podcast, but how do you suggest that we make jobs and take consideration into making sure that AI doesn't take all the jobs?
Thanks. Several things,
[00:28:24] Fei-Fei Li: Hala. First of all, why do we have jobs? It's really important to think about it. I think jobs is part of human prosperity because we need that to translate into financial reward so that we have the prosperity that our family and we need. It also is part of human dignity. It's beyond just money.
For many people, it's the meaning of life and Self respect. So from that point of view, I think we have to recognize jobs shift throughout human history, technology, and also other factors creates, destroys, morphs, transforms jobs. But what doesn't change is the need for human prosperity and human dignity.
So I think when we think about AI and its impact in jobs, it's important to go to the very core of what jobs are and means and what technology can do. So when it comes to, say, human dignity, for example, I do a lot of healthcare research with AI, and it's so clear to me That many of the jobs that our clinicians and healthcare workers do are part of humans caring for humans and that emotional bond, that dignity, that respect can never be replaced.
What is also clear to me is that American healthcare workers, especially nurses, are over fatigued, overworked, and if technology can be a positive force to help them, To help them take care of patients better to reduce their workload, especially some of the repetitive, thankless work like constant charting or walking miles and miles a day to fetch pharmacy medicines and, and all that.
If those parts of the job, the tasks can be augmented by machines. It is really truly intended to protect the human prosperity and dignity. But augment human capabilities. So from that point of view, I think there is a lot of opportunity for AI to play a positive role. But again, it depends on, first of all, it depends on how we design AI.
In my lab, we did a very interesting research. We were trying to create a big robotics project to do a thousand human everyday tasks. But at the beginning of this project, It was very important to us that we are creating robots to do these tasks that humans want help. For example, buying a wedding ring.
Even if you have the best robot in the world, who wants a robot to choose a wedding ring or opening Christmas gift? It's not that hard to open a box, but the human emotion, the joy, the family bond, the moment is not about opening a silly box. So we actually ask people to rank for us thousands and thousands of tasks.
And tell us which tasks they want robots help, for example, like cleaning toilet. Everybody wants robots help. So we focus on those tasks that humans prefer robotic help, rather than those tasks that humans care and want to do themselves. And that is a way of thinking about human centered AI. How do we create technology that is beneficial, welcomed by humans, rather than, I just go in and tell you.
I'm using robot to replace everything you care about. Another layer, just to finish this topic, is policy layer. Like, economic, social well being is so important, and technologists don't know it all, and we shouldn't feel we know it all. We should be collaborating with civil society, legal world, policy world.
Economists to try to understand the nuance and the profoundness of jobs and tasks and A. I. 's impact. And this is also why our human center A. I. Institute at Stanford has a digital economy lab. We work with policymakers and thinking about these issues. We try to inform them and provide information to help move these topics forward in a positive way.
[00:32:57] Hala Taha: you have three aspects to your human centered AI framework, right? So AI is interdisciplinary, AI needs to be trying to make sure that we have human dignity and using it for human good. And then there's also one about intelligence. Can you break down your three?
pillars of your human centered AI framework?
[00:33:17] Fei-Fei Li: The three pillars of the human centered AI framework is really about thought leadership in AI and focusing on what higher education institutes like Stanford can do. One we talked about is recognizing the interdisciplinary nature of AI, welcoming the multi stakeholder studies, research and education, uh, policy outreach to make sure that AI is embedded in the fabric of our society today and tomorrow in a benevolent way.
The second one is what you said is focusing on augmenting humans, creating technology that enhances human capability and human well being and human dignity rather than taking away. The third one is about continue to be inspired by human intelligence and develop AI technology that is compatible with humans.
Human intelligence is very complex. It's very rich. We talked a lot about emotion, intention, compassion, and, uh, today's AI lacks most of that. It's pretty far from that. Being inspired by this can help us to create. And also, by the way, there's another thing about today's AI that is far worse than humans.
It draws a lot of energy. Humans, our brain works around 20 watts. It's like dimmer than the dimmest light bulb in your house. Yet we can do so many things. We can create the pyramid. We can, you know, come up with E equals MC squared. We can write beautiful music and all that. AI today is very, very energy consuming.
It's, uh, It's bulky, it's huge. So there's a lot in human intelligence that can inspire the next generation of AI to do better.
[00:35:10] Hala Taha: Every time I have an AI episode, I feel like I learned so much that I didn't really realize before. We've had conversations with other people on the show about how a lot of people are scared of AI getting apex intelligence, that it's going to be so much smarter than humans, going to take over the world, it's going to control humans.
Do you have any fears around that?
[00:35:30] Fei-Fei Li: I do have fears. I think who lives in 2024 and don't have fears? And as a citizen of the world, I think our civilization, our species is always defined by the struggle of dark and light. And by the struggle and good and bad, we have incredible benevolence in our DNA, but we also have incredible badness in our DNA and AI as a technology can be used by the badness.
So from that point of view, I do have fear. The way I cope with fear is try to be constructively helpful is try to advocate for the benevolent use of this technology and to use this technology to combat the badness. At the end of the day, any hope I have for AI is not about AI, it's about humans. To paraphrase Dr.
King, the arc of history is long, but it does bend towards justice and benevolence in general. But to come down from that abstract thinking, I think we have work to do. Because if AI is in the hands of bad actors. If AI is concentrated in only a few powerful people's hand, it can go very wrong. We don't need to wait for sentient AI.
Even today's car, imagine there is a bad person who is in charge of building 50 percent of America's car, and that person just wants to make all the car brakes malfunction. Or add a sensor and say, if you see a pedestrian, run it over. Actually, today's technology can do that. You don't need sentient AI.
But the fact that we don't have that dystopian scenario is first of all, human nature is buying large goods. You know, our car factory workers, our business leaders in building cars, nobody thinks about doing that. We also have laws. If someone is trying to do harm, we have societal constraints. We also try to educate the population towards.
Good things. Right? So all this is hard work and we need that hard work in AI to ensure it doesn't do bad.
[00:37:55] Hala Taha: I just want to give an example that when I was talking to Stephen Wolfram, because the interview is fresh in my head, and he said something that made me feel a little bit at ease with AI and the fact that it could get really smart.
He said, we're living in AI. We live in nature. Nature is so complex. We can't control it. It has simple processes that are really, really complex. We can predict it all we want. We'll never really know what nature is going to do. And already we live in a world where we're interacting with nature every day and we have to just deal with the fact that we don't control it and it's smarter than us to a degree.
And he's like, that's what maybe AI will be like in the future. It will be there. It will be its own system. What are your thoughts on that?
[00:38:36] Fei-Fei Li: That's a very interesting way to put it. Okay. First time I heard that. I like his way of saying that humans in the face of complexity and powerful things. That we still have a way to cohabitate with it.
I don't agree nature is AI in the sense that nature is not programmable. And I don't think nature has a collective intention. It's not like the earth wants to be a bigger earth or bluer earth or, or, you know. So from that point of view, it's very, very different. But I appreciate the way he says that. And I also think using his analogy, we also live with other humans.
And there are humans who are more stronger than us, smarter than us, do better, whatever, than us. But yet, by and large, our world is not everyone killing each other, by and large. Now, this is where we do see the darkness. And this has nothing to do with AI. Human nature has darkness and we harm each other.
And the hope is, it's not just the hope, the work is that when we create machines that resemble our intelligence. We should prevent it to do similar harms to us, to each other, and try to bring out the better part of ourselves.
[00:39:59] Hala Taha: As we wrap up this interview, I wanted to ask you a couple of questions.
So first off, You're talking to a lot of young entrepreneurs right now and people who want to be entrepreneurs. What's your advice to them about how to embrace this AI world?
[00:40:12] Fei-Fei Li: So first of all, I hope you read my book, The Worlds I See, because the book is written to young people for young people. It's a coming of age of a scientist, but the true theme of the book is finding your North Star, is finding your passion.
And believing that against all odds and chase after the North star. And that is the core of what entrepreneurship is about is that you believe in bringing something to the world and against all odds, you want to make it happen and that should be your North star in terms of AI. It's a incredibly powerful tool.
So it depends on what business and products you're making. It either can empower you or it's a essential part of your. Core product or it keeps you competitive. It's so horizontal that for most entrepreneurs out there, if you don't know anything about AI, it is important to educate yourself because it's possible that AI will play either in your favor or in your competitor's favor.
So knowing that is important.
[00:41:24] Hala Taha: I'm just going to ask you one last question. And this is really about visioning. Let's vision a world 10 years from now, 2034, where there's human centered AI. And let's also try to visualize a world 10 years from now where maybe it's not human centered AI.
Maybe it got in the bad hands of some folks. Let's talk about those two worlds, and then we'll close it out.
[00:41:49] Fei-Fei Li: The world that's human centered AI, I think it's not too far from at least the North America world we live in, even though I know we're not perfect, is that we still have a strong democracy. We still believe in Individual dignity and by and large free market capitalism that we are allowed as individual to pursue our happiness and prosperity and respect each other and AI helps us to do better scientific discovery to have self driving cars to help people who can drive.
Or, you know, reduce traffic to make life easier, to make education more personalized, to empower our teachers and healthcare workers to discover a cure for diseases, to alleviate our aging population problems, to make agriculture more effective, to find climate solutions. There is so much AI can do in the world that we still have the good foundation.
Now, the dystopia world is, AI can be used as a bad tool to topple democracy. Disinformation is a incredibly harmful way of harming democracy and the civil life we have right now. If it's completely concentrated in power, whether it's state power, individual power, it makes the rest of the society much more subject to the.
will and possibly wrath of that power, whether it's AI or not. We have seen in human history that concentrated power is always bad and concentrated power using powerful technology is not a recipe for good.
[00:43:45] Hala Taha: Well, Dr. Li, I'm so happy we have somebody like you who's helping us to navigate the AI world, who's also helping to shape the AI world in a way that hopefully is going to be good for humans.
Please let us know where we can learn more about you and everything that you do. Thank you, Hala.
[00:44:00] Fei-Fei Li: Thank you for promoting my book and please constantly check in with Stanford Human Center AI Institute newsletter and website.
[00:44:08] Hala Taha: Amazing. We'll stick all those things in the show notes. Dr. Li, thank you for joining us on Young and Profiting podcast.
[00:44:13] Fei-Fei Li: Hala. Hala.
Yeah, fam, it is clear that AI has the potential to be a powerful tool. But it's also important to keep things in perspective. Remember the quote that Dr. Lee shared, the most advanced computer AI algorithm will still play a good chess move when the room is on fire. For the time being, we humans have much more fluid, organic, and contextual understanding of ourselves and our own thoughts and emotions.
And AI still cannot create in the way that humans can create.
There's so much potential for good when it comes to AI, like those rescue robots and cleaning robots that Dr. Li described. Not to mention the way that some overworked professions like nursing, for example, could be greatly improved by technology that could help them And if used well, AI has the capacity to bolster not only our capabilities, but also our prosperity and our dignity. But this will depend in large part on us and whether we can encourage and foster what Dr. Lee calls human centered AI. The biggest risk regarding AI is likely not AI turning evil, but AI being deployed by bad people.
But if you think about it, cars, guns, and other things like that can all be abused and misused and they are. But that's why we have laws and policies and social norms that help guard against those things. And hopefully that will be the same with AI, we'll have regulations around it to help protect us from the bad things that can happen from bad people who use AI.
And that will especially be more likely to happen if Dr. Li and others like her can carry the day. And if they can, then perhaps, and hopefully, we will live in that first alternative future universe that Dr. Li described. The one where AI helps us improve scientific discovery. Develop amazing self driving cars, personalize education, and ultimately help us lead more comfortable and fulfilling lives.
Thanks for listening to this episode of Young and Profiting Podcast. Every time you listen and enjoy an episode of this podcast, share it with your friends and family. Maybe someday an AI bot will be able to do that for you. But until then, we really do depend on you to share this podcast by word of mouth.
And if you did enjoy this show and you learned something new, then please drop us a five star review on Apple Podcasts. I read these reviews every single morning. It makes my day. So if you want to make my day, go take two minutes and write a positive five star review on Apple Podcasts.
And maybe I'll shout you out on an upcoming episode. And if you prefer to watch your podcasts as videos, you can also find all of our episodes uploaded to YouTube. Just look up Young and Profiting You can also find me on Instagram at Yap with Hala or LinkedIn.
My name is Hala Taha. You can just search for my name. And before we wrap, I always have to give a big thank you to my incredible Yap production team. You guys are so hardworking. You're so talented. There's too many of you to shout out now. The team is growing so fast, but thank you for all that you do on my podcasts, on the other network podcasts.
You guys are amazing. Thank you so much. And this is your host, Hala Taha, AKA the podcast princess, signing off.
Episode Transcription
Enter your name and email address below and I'll send you periodic updates about the podcast.